What if every interaction with your brand felt like it was designed specifically for you? This is the promise of the “segment of one,” the bedrock of today’s personalized commerce. Propensity modeling, leveraging the power of AI, makes this a reality by predicting individual customer behaviors – be it a pending purchase, a risk of leaving, or their preferred way to connect. This foresight enables hyper-personalized targeting, driving engagement and maximizing ROI through perfectly timed and relevant communications. Are you asking: How does propensity modeling reduce churn and keep valuable customers loyal? Can I really fine-tune my marketing budget by targeting only those most likely to convert? And crucially, how can I achieve “segment of one” personalization across my entire customer base? You’ve come to the right place – this blog will guide you.
What Is a Propensity Model?
A propensity model is a machine learning algorithm that predicts the likelihood of a customer taking a specific action, such as making a purchase, unsubscribing, or becoming inactive. It analyzes historical data like purchase behavior, browsing activity, and engagement to assign probability scores to users. This helps marketers prioritize efforts on high-value segments and reduce spend on low-probability ones.
How do we use propensity modeling to predict customer behavior?
Customer propensity models predict how likely each user is to take a specific action, enabling ecommerce brands to create smart, personalized campaigns. For example, users with high purchase propensity can receive tailored recommendations, while low-propensity users get stronger incentives. Campaigns can be segmented by score, triggered by real-time behavior, and delivered via preferred channels. This targeted approach improves conversions, repeat purchases, and customer lifetime value, while reducing wasted spend, making every campaign more efficient and profitable.
Types of Propensity Models
Propensity modeling offers a variety of predictive frameworks tailored to specific business goals. Here are the most impactful types:
1. Propensity to Purchase/Convert
This model predicts the likelihood that a customer will make a purchase in a given time frame. It helps prioritize high-intent users and craft targeted campaigns that nudge them toward conversion.
2. Propensity to Churn
This model identifies customers likely to stop engaging or leave. Businesses can use it to proactively run win-back campaigns, deliver loyalty rewards, or address potential dissatisfaction before it’s too late.
3. Propensity to Engage
This model estimates the probability that a user will interact with content such as emails, push notifications, or app messages. It helps marketers optimize timing, content type, and channels for maximum engagement.
4. Customer Lifetime Value (CLV) Modeling
While not a propensity model in the traditional sense, CLV modeling uses similar predictive techniques to forecast a customer’s long-term value. This insight helps businesses allocate resources to high-value segments and tailor retention strategies accordingly.
Each model serves a unique purpose and can be used in combination to drive end-to-end lifecycle marketing, from acquisition to retention and reactivation. The models are robust enough to treat each client in a different way according to their needs and find out what works best to predict churn for your business. You can follow the diagram below for a better understanding.
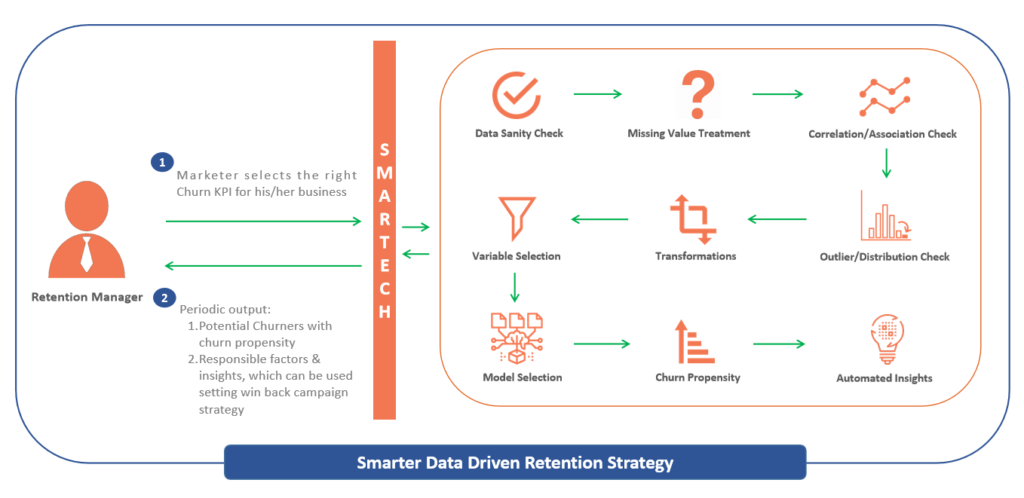
Propensity Modeling Use Cases in Ecommerce
Propensity modeling is being adopted by top ecommerce and digital-first brands to achieve a wide range of outcomes, from increasing repeat purchases to reducing churn. Here are some real-life use cases:
1. Predict Conversions and Drive Repeat Purchases
Amazon uses advanced propensity models to recommend products based on browsing and purchasing behavior. These recommendations significantly drive repeat purchases and contribute to over 35% of its revenue. By suggesting relevant products with high conversion likelihood, Amazon keeps customers engaged and loyal. Netcore leverages propensity modeling and AI to deliver precisely targeted product recommendations.
2. Prevent Uninstalls and Dormancy
Spotify predicts disengagement using listening patterns and interaction frequency. It then re-engages users with custom-curated playlists like “Discover Weekly,” personalized to individual tastes. This predictive approach increases listening time and reduces churn.
3. Churn Prediction and Customer Retention
Netflix uses churn propensity scores to personalize its content interface and recommendations. By showing users what they’re most likely to watch next, Netflix reduces the risk of inactivity and keeps subscribers engaged, directly impacting retention and customer lifetime value.
4. Cross-Sell and Upsell Optimization
Myntra, a fashion ecommerce brand, uses ML models to cross-sell complementary products such as shoes or accessories with viewed clothing items. By understanding the user’s style and purchase behavior, Myntra improves average order value and shopping experience.
5. Customer Lifetime Value Targeting
Sephora utilizes CLTV predictions to prioritize high-value customers in its Beauty Insider program. These customers receive personalized offers, early access to product launches, and exclusive perks, leading to higher repeat purchase rates and deeper brand loyalty.
6. Lifecycle and Behavioral Targeting
Target famously applied propensity modeling to predict major life changes like pregnancy by analyzing subtle shifts in purchase behavior. This allowed them to deliver extremely relevant offers at just the right time in a customer’s life, increasing engagement and loyalty.
7. Geo-Based Offers and In-Store Conversion
Starbucks combines geofencing with propensity modeling to send time-sensitive offers via its app when users are near a store. This drives impulse visits and increases conversions from nearby foot traffic.
These examples show how predictive models are helping brands not just segment smarter, but act faster and more precisely—maximizing engagement, revenue, and customer satisfaction.
Predictive Propensity Modeling: Driving Ecommerce Growth
1. Model to Calculate Customer Lifetime Value (CLV)
Propensity modeling improves CLV predictions by factoring in future buying behavior, retention likelihood, and spending patterns. Ecommerce brands can use these insights to segment customers by predicting long-term value and personalizing marketing accordingly. This helps in budget allocation, maximizing average order value (AOV), and increasing repeat purchases, all of which contribute to sustainable growth.
2. Churn Propensity Models: Identifying At-Risk Customers
By detecting subtle behavioral shifts—like reduced browsing time, longer gaps between purchases, or disengagement—churn models flag customers likely to leave. Ecommerce businesses can launch timely retention campaigns, send re-engagement nudges, or offer loyalty perks to reduce churn, safeguard CLV, and boost retention rates.
3. Optimize Marketing Spending with Smart Segmentation
Propensity models identify which campaigns may underperform with specific customer cohorts. Instead of mass messaging, brands can concentrate resources on high-intent segments, improve ROI, and reduce CAC (Customer Acquisition Cost). This ensures every dollar spent is aligned with conversion probability and relevance.
4. Unlock Hidden Opportunities
AI-driven insights can spotlight customer cohorts previously overlooked—those with a high likelihood of conversion or repeat purchases. These “hidden gems” can be targeted with curated offers, expanding your customer base and increasing conversions and average basket size without extra ad spend.
5. Deliver Better CX with Preferred Channels
Propensity models determine the best communication channel for each user—email, push, WhatsApp, SMS—based on engagement history. This improves open and response rates avoids spam fatigue, and enhances customer experience, ultimately leading to higher satisfaction and long-term loyalty.
Advanced ML Applications: Precision Targeting & Revenue Growth
Machine learning adds an intelligent layer to predictive modeling, allowing ecommerce brands to deliver next-best recommendations and proactively engage each customer:
- Cross-selling: AI identifies category-agnostic suggestions based on interest overlap, e.g., showing wireless Bluetooth headphones with sneakers for fitness buyers, boosting AOV and product discovery.
- Up-selling: Pretrained models surface higher-end or complementary items in the same category, increasing premium conversions.
- Customer Lifetime Value (CLTV): Predicts who will be your most valuable customers, helping allocate retention vs. acquisition resources effectively.
- Customer Engagement: Anticipates when a customer may drop off and adjusts campaigns to keep them engaged.
- Campaign Recommendations: Analyze past campaign performance to auto-generate optimization insights, reducing trial-and-error costs.
- Lookalike Modeling: Find new prospects who mirror your best customers, improving acquisition efficiency.
- Awareness & Engagement: Identifies high-propensity users for top-of-funnel nurturing.
- RFM Modeling: Scores users based on Recency, Frequency, and Monetary value to target high-yield segments with surgical precision.
- GeoFencing: Targets users within physical proximity to stores or events, boosting footfall and localized engagement.
- Channel-Specific Behavior Prediction: Helps deliver channel-optimized content for maximum engagement and conversion.
Conclusion
Propensity modeling transforms ecommerce marketing from broad-based campaigns into precision-guided, revenue-optimized journeys. By predicting what each customer will do next—whether it’s buying, churning, or engaging—brands can 3x their ROI, increase CLTV, and build lasting customer relationships. The result? More repeat purchases, higher conversion rates, and sustained business growth.
Ready to unlock the power of predictive insights for your ecommerce business?
Book a personalized demo with our experts to see how we can curate a tailored propensity modeling strategy specifically for your business and start achieving predictable, exponential growth.