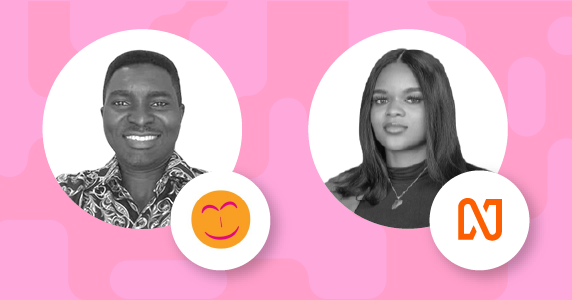
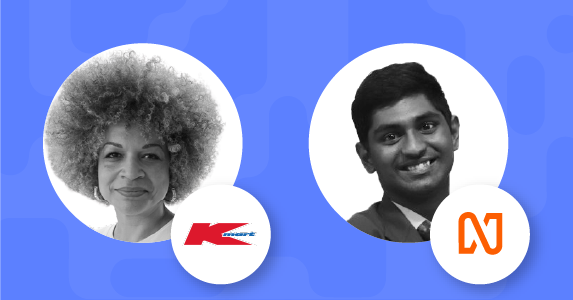
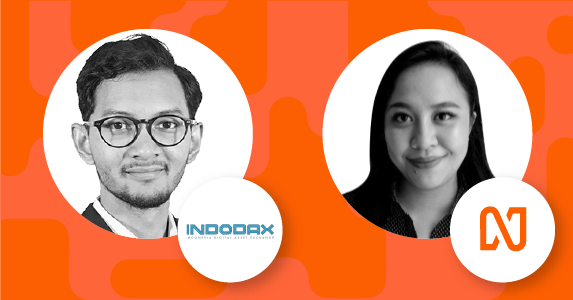
In this session of The Martechno Beat Podcast, we are joined by Vrinda Aggarwal – Lead, Customer Lifecycle Management at Join Ventures – to talk about how AI/ML in marketing is evolving and benefiting the new age marketers.
We also discuss various things marketers need to keep in mind when trying to utilize the power of AI and ML to boost customer engagement and conversion.
IGP is an online retailer of personalized, floral, gourmet and handmade gifting products. The company is headquartered in Mumbai, India and has offices in India, Singapore and the United States. IGP is owned and operated by Join Ventures.
Shailesh Mourya (00:00:07)
Hello everyone, welcome to another super insightful episode of Martechno Beat, a specially curated podcast series powered by Netcore Cloud. On this podcast, you can gain cutting edge insights from the leading marketeers on all things related to customer engagement, acquisition, and retention. I’m your host, Shailesh Mourya and I’m joined by a very special guest today, Vrinda Aggarwal. She is the Lead for customer Lifecycle Management at Join Ventures. Hey, Vrinda, how are you doing today?
Vrinda Aggarwal (00:00:38)
Hi Shailesh, it’s nice to be here.
Shailesh Mourya (00:00:41)
Vrinda, it’s my pleasure to have you on this podcast. Thank you for joining. Guys, Vrinda comes with a very rich experience of eight-plus years in various roles in digital marketing. Virendra, why don’t you want to quickly tell our audience about your career journey so far? And, yes recently you completed an advanced program in AI powered marketing from IIM Calcutta. How was your experience going back to the classroom?
Vrinda Aggarwal (00:01:09)
Thanks Shailesh. So that experience was amazingly fun and stressing at the same time. I was back in the classroom after a decade and so a mixed bag of experience but definitely an enlightening journey and getting me ready for the next phase of my career In terms of that career, so far I am working now with Join Ventures for the last five years and I take care of the customer retention, lifecycle improvement and overall customer touchpoints, which goes out right from when the customer is doing the first visit on site till the time they transact and beyond that. Before that I have been a startup person throughout and I have worked in industries ranging from hospitality to restore bills to within the Defense and Homeland Security. That was my first role and I have done – I have worked on the content marketing side with my first job being the engagement and activation persons to contemplate the workshops and the case materials and the textbooks defense and Homeland Security practice. Then to the acquisition marketing side of things on digital were the next two roles with the various startups. And now on the retention piece with Join Ventures.
Shailesh Mourya (00:02:41)
Perfect. So it seems you have got a very deep experience about managing the entire life cycle of the customers and that’s where I’ve experienced the kind of work that you’re doing. I would like to congratulate you on the recent success that IGP.com has achieved, the kind of communication that you guys are doing is awesome. Could you tell our audience a bit more about Joint Ventures, how it started, what is the target audience you’re trying to cater to and what has been the growth story so far?
Vrinda Aggarwal (00:03:15)
Sure. Thanks for the kind words. So Join Ventures is the house of D2C brands and we have three brands under the umbrella: IGP.com, which is our celebratory ecosystem brand in the mass space. And then second is interflora.in, which is our lifestyle brand for flowers and special hampers and events and wedding decor with the mostly premium imported luxury flowers from across the globe, then IGP for business, which is our corporate gifting arm with the solution changing from one time, corporate gifts for the events like Diwali, New Year, employee Joining kids etc., to white label solutions for large Fortune 500 companies to be used internally as the one-point gifting total employee reward system and recognition software.
Shailesh Mourya (00:04:13)
Vrinda, I’m actually a — personally an IGP customer. I-I love the kind of collection you guys have, especially in the personalized gifting space, I’ve been using it very often. I’ve been using IGP to send certain gifts to my Clients, to my friends and the feedback has always been very positive.
Vrinda Aggarwal (00:04:38)
Wow, happy to hear that.
Shailesh Mourya (00:04:39)
Yeah, and kudos to the entire IGP team for that. So, now let’s get started with our topic of discussion today, which is AI/ML in marketing. Vrinda, over the last few years, the development of AI/ML has skyrocketed and doing certain tasks for marketeers, which would take hours earlier, is happening in minutes. And it is a system which is taking care of these basic tasks which required a lot of effort earlier. And now with that, because customers’ needs and expectations are changing very fast with the interactions that they’re having with different brands, more and more customers are expecting a very consistent and very evolved experience with the brand in whatever touch points that they are interacting with—with the brand.
Vrinda Aggarwal (00:05:30)
That’s really very true. That’s very true.
Shailesh Mourya (00:05:33)
So according to you, what is the role that AI/ML plays for marketeers to achieve that? And before we get there, why don’t you tell our audience what AI/ML in marketing, according to you and what it actually does?
Vrinda Aggarwal (00:05:51)
OK, so, I’d like to quote a blog—a snippet from Salesforce here, because I think that accurately bends down in… in a very understandable language. So, AI/ML simulates how humans think and help marketers deliver a personalized customer experience with the scale and efficiency of a machine. And the best part is that it learns from the experience of every interaction on its own and then improves upon the messages which are getting delivered. And that entire learning process mimics just how a human being learns about —
Shailesh Mourya (00:06:28)
Yeah,yeah. Can you share some examples about how AI/ML actually works?
Vrinda Aggarwal (00:06:32)
Shailesh Mourya (00:07:52)
Yeah, so you are saying that a job which required effort from the agency, (you had to brief somebody to do multiple rounds of meetings and then come up with various design versions for the logo) is getting tackled by the system completely?
Vrinda Aggarwal (00:08:17)
OK, so ok, so, that’s a debate: how much of a human versus machine can go in? But—So, so, the way I see it, logo builder, if you’re a small-scale startup and you can’t really afford the top dollars on the agency, then this tool is heavenly for you. If you are — If you are a client or if you are a company with huge pockets and is definitely looking to create one of its own kind in a complete industry or a first-mover advantage, agencies are still the go.
Shailesh Mourya (00:08:42)
Yeah, absolutely. I think nothing can beat human intelligence. But, I would like to stick to the topic and would like to understand from you a bit — from you a bit more about the different tools which are out there in the market that you have covered. But, why do you think marketers actually need to use these tools, and why AI/ML is such a buzzword in the marketing fraternity?
Vrinda Aggarwal (00:09:07)
So — See, AI/ML, at a conceptual level, or as a mathematical theory has been around for, you know, longer than sixty years now, but I guess it’s now that the computational power in the hands of common man is catching up, that you can run those models on your own. Otherwise, even five years ago, you really needed that system sitting in with bigger companies only to be able to do that much of the computation and, you know, feed the data and get the results out as required to be used in everyday parlance. Secondly, the total amount of data that the marketing companies are now generating is — has just, you know — has just doubled every, you know — has grown, in fact, exponentially every year and that becomes, you know, a cornerstone for any AI/ML to run properly. And third is that the companies have also become very sophisticated in how they’re storing that much amount of the data and how quickly they can, you know, have a tap into that data. Earlier it used to be that data warehouses were sitting in silos at the company premises and people had to physically move to be able to tap into their data. It’s not so anymore with the advent of cloud technologies and the huge drive spaces available on cloud also for the companies to run simultaneous operations. So, all these things combined have enabled the market now to be AI/ML-ready and the final push has come with this entire ecosystem in marketing where the third party cookies are getting deprecated from, you know, the likes of Google, the Metaverse and then your Android technology. And companies are—
Shailesh Mourya (00:10:51)
This development is actually putting a lot of marketeers under a lot of stress yeah.
Vrinda Aggarwal (00:10:57)
Stress, that’s true, because it’s a different shift. It’s a tectonic shift. All together how do you see marketing? How do you see your acquisition strategy and even your remarketing and retention strategy? So, with this shift, first-party data is gaining a lot of spotlight, and first-party data, because of the size, volume, and the — veracity required, can be properly used only with AI/ML techniques. It’s not humanly possible now to run it through, you know, the old-school Excel and — or even stuff like a data visualization page.
Shailesh Mourya (00:11:32)
You actually need a system and proper algorithms to manage these data points. I would like to also touch upon a very famous concept now which is zero party data, which — which is the data, which a customer himself is sharing with the brand and that is also getting a lot of momentum because it is one of the most important data that a brand can use to further leverage the power of personalization in whatever communication they’re doing. Imagine you combine the customer’s preference data that he is sharing with his past transaction data and then also club it with the recent behavior on the website or on the app. By combining this you can create certain predictive segments, by the way, which Netcore also has which we can talk about a little bit more about later. The overall results of the campaigns can — can be much, much better as compared to what would be in a plain vanilla campaign.
Vrinda Aggarwal (00:12:36)
That’s absolutely true. That’s completely true, Shailesh.
Shailesh Mourya (00:12:42)
Yeah, yeah. So Virendra the automation in omnichannel marketing through customer engagement platforms has been there for many years now, what according to you has changed in the new AI/ML based platforms?
Vrinda Aggarwal (00:12:56)
OK, so, most of the automation platforms that we used in the past in marketing, they were rule-based engines, so, say you could, you could say that if the customer is doing X and Y but not a Z then a set of communication has to go out. The customer has an X but not Y and then there’s a different kind of communication to be going out.
Shailesh Mourya (00:13:22)
So, all of these are marketing ideas, finally.
Vrinda Aggarwal (00:13:24)
Market you define the inputs, definitely, from their understanding of the data and the customer profiling. And then accordingly, tweaking the communication. Now the problem with the rule is — is that at one goal you can — at maximum you can eyeball or, you know, you can analyze up to, say, twenty attributes —
Shailesh Mourya (00:13:44)
That’s right.
Vrinda Aggarwal (00:13:48)
or twenty data points for each customer for you to come up with a data set. This means that most of the time the segmentation which we were arriving at can be, you know, seven, eight, up to fifteen, twenty in bigger companies. But beyond that it becomes a little hard task to follow through then, to see that.
Shailesh Mourya (00:14:02)
It would be humanly impossible to go deep down further than that.
Vrinda Aggarwal (00:14:06)
Exactly. And similarly, for the system also, probably a journey mapper can handle you placing five conditions in a single journey or ten conditions, but beyond that you can’t go. So, that was the entire ecosystem of automation, which served us very well when we were looking to do personalization of the content to the customers, but not hyper-personalization, and you were not treating each customer, you were treating each customer impact basis the sum total of their past behaviors and you expected them to continue with the same mode.
Shailesh Mourya (00:14:38)
Which may not always be true.
Vrinda Aggarwal (00:14:40)
Which is not true even in human behavior. Now, what the AI/ML-based platform does for you very beautifully, is they take into account your past behaviors, but rather than segmenting the data only, they’ll also predict what is the most likely action, and, according to you, you can tweak your conditions and communications. So, no longer are you designing your communication patterns, basis the rules which define the past behavior and segments, but you’re tweaking your communication on the most likely outcomes.
Shailesh Mourya (00:15:12)
Can you give some examples here, Vrinda?
Vrinda Aggarwal (00:15:15)
Sure. So, for example, you have two customers who have done two transactions with the brand. Now, if you were having a rule-based system taking input from say ten other attributes, most likely they would plug these two customers in one bucket, saying that on the way to two, it might become a loyal customer, say, the rest of the parameters are all same, except the kind of network connectivity that they use. So, one is on a low-end mobile phone
Shailesh Mourya (00:15:41)
Bandwidth, yeah.
Vrinda Aggarwal (00:15:51)
2G spectrum coming from a Tier 3 city and another one is on a high-speed Wi-Fi connection coming from a — coming from the same city. Now, when you run predictive segments, What they will do… they will take into account the past behavior and these kinds of data addresses of these two customers, but they will also understand that is how a customer, similar to these conditions, from the flats of datasets that we already have compiled from other customers, behave – and basis that, it will predict the likely outcome. Say we are looking for a first transaction outcome, so, it might come differently that the customer with a high connectivity is more likely to transact in the next ten days again. But a customer with a low connectivity or a 2G might transaction say after two months.
Shailesh Mourya (00:16:27)
I think these models can also help marketers identify the — what is the ticket size that most — most likely that customer is going to buy from the brand and accordingly offers can be tweaked. I may want to run one offer which is for my high-end customers with the ticket size say ₹5000, whereas the other offer could be primarily sub ₹1000
Vrinda Aggarwal (00:16:51)
Completely — completely totaled, in fact, the most used example, or the most — most widely accepted use case now for this, is when you apply for a loan, say for Paisabazaar.com, a prediction algorithm actually intimates the banks for which you are applying that what is the likely profile of that customer? And accordingly the type of offers, the type of lending rates that you will see… they will get changed. So, it’s a real-time elbow — AI/ML elbow running behind it, which is classifying the customers into different categories and accordingly, the outcomes are getting great.
Shailesh Mourya (00:17:26)
Yeah, that’s correct. Since you mentioned AI/ML features, let me also talk about a very powerful feature of Netcore’s Raman, which is our own AI engine, which can be very useful for brands. The feature name is predictive segments. So, predictive segments, what it does is it —
Vrinda Aggarwal (00:17:44)
We have been using it now for about a year.
Shailesh Mourya (00:17:46)
A year. Yeah, it has — it has been giving great results for e-commerce brands. So, what predictive segment does is that it predicts future actions of any segment of audience, basis past data points that are already available. It could be its past transaction history or users’ behavior on your website or on the mobile app, and then the prediction scope can be defined by the marketeer himself. When he’s running a campaign, the objective of the campaign might be different each time, right? So, the marketeer can define what the objective is and then Raman can do its prediction magic and comes up with certain segmentation. These segments could be something like, say, users who are more likely to purchase in the last—next seven days, or users who are most likely to convert to any cross-sale campaigns because of their past transaction history, or something else like — which is very true for app-first brands who are the users who are likely to uninstall the app; which is called churn prediction model in Raman. Raman gives you three different segments for each of these segments that you define, which are these micro-segments based on propensity. Now these propensities are something like users who are most likely to perform a certain activity, which is the most — highest probability of the action. Users who are in the ‘fairly likely’ bucket, and finally users who are in the ‘less likely’ bucket, where the confidence level is very low. So, these are the kind of features that are in-built in our Raman capabilities that we have.
Moving on, Vrinda, what are some of the use cases that you think AI/ML can power marketeers, especially in the e-commerce industry, because IGP is operating in that function, it will be great if you could share your experience and your understanding so far on this topic.
Vrinda Aggarwal (00:19:40)
Some of them.
Shailesh Mourya (00:19:41)
Use cases that you think AI/ML can power marketers, especially in the e-commerce industry. Because IGP is operating in that function, it will be great if you can share your experience and your understanding so far on this topic.
Vrinda Aggarwal (00:19:58)
Sure. So, the use cases in any industry, and definitely in the e-commerce industry, abound where AI/ML is getting juiced, also, quite a lot. So, for example, what content would drive a better engagement for a given cohort? Right? From subject line suggestions — which is available, to the content pieces, what kind of pictures, and the placements? Now all can be done with a more data-driven approach rather than just a visual aesthetic approach. Then — And, in fact, much better than the only A/B testing or split testing experiments that—that we used to do earlier. But customers need a nudge to, or incentive to, convert in the next three days, say, or the uninstall of the app example for the predictions that you put
Shailesh Mourya (00:20:47)
Yeah, that recovery.
Vrinda Aggarwal (00:20:48)
All of these are very everyday use cases which were earlier getting solved by certain rule-based and inherent understanding of the customer data set, and now, in a more, more real environment with hundreds of data points getting used to coming up with those predictions. So, the way I see it, any company can define any — every business problem can be defined into AI/ML-based solutioning problem
Shailesh Mourya (00:21:14)
Yeah, that’s interesting.
Vrinda Aggarwal (00:21:14)
The approach — can be — yeah — two kinds: one way is that you have a bottoms-up approach, so, ‘bottoms approach’ typically means that you have an available data set already collected with you. You run different kinds of models and then, based on the outcomes, then you define the business goals. So, a typical example for it would be on predictive segments of, say, uninstall/install/most likely to convert users on the app in the next seven days. So, there the data is already sitting for the thousands of people who have done the same exercises at different points of time. You are just running that predictive model to understand what profile of the users are likely to do a particular action and, on the basis of that probability, you can tweak your communication with the send-out incentives, you can define the communication journey, or you can define different touch points to lower down all those negative factors. Now, another one is a top-down approach. Now ‘top-down approach’ means that you have a defined business problem sitting for you. Then you — based on that problem, you define what is a data set required for you to take an informed decision or take informed action for that business problem solution. And then you set about collecting that data and finally, you run AI/ML and then an algorithm, basis that.
Shailesh Mourya (00:21:20)
Can you give some examples between what is the top down approach versus bottom bottom sub approach?
Vrinda Aggarwal (00:21:34)
So, for example, the bottoms-up approach we discussed right away for, you know, the app uninstalls and churn. Another one which mostly is getting used in e-commerce websites is the recommended shopping list for the customers. So, what they typically are doing, is – the transactional data which has been sitting in the warehouse now for millions of customers for them — analyzing and modeling that data to identify the different profiles of the current shoppers living on the website and then provide a recommendation list. Which are similar items, recommended items, most frequently purchased together, or the things that ‘You may like this as well’. All of these are your bottoms-up approved. A top-down approach would be, you know, something like fraud detection, which happens in the credit card industry. So, credit card companies were, well, they were rolling out, even in India, so, they were always on to the consideration of how to detect a fraud transaction versus a general transaction.
Shailesh Mourya (00:23:18)
Yeah. OK.
Vrinda Aggarwal (00:23:28)
So credit card. Companies were well, whenever rolling out, even in India, so they were always on to consider how to detect a fraud transaction versus a general transaction.
Shailesh Mourya (00:23:40)
Triangle yeah.
Vrinda Aggarwal (00:23:41)
Because customer behaviors, spending patterns, traveling patterns… Everything keeps changing. So, rule-based generally used to have high false positives rather than actual positives. So, AI/ML helps to make it — to comment at a very accurate level. Because it not only takes into account the past data, but also talked about how our customer lifecycle generally progresses, and that became the fraud detection tool which was with the credit cards, so. And I’m sure most of the listeners would have experienced suddenly your card which is getting fired for two thousand — two thousand bucks, if it gets fired for twenty thousand bucks, sometimes they would get a call, but the frequency of those calls has gone down because credit card companies have also learned how to profile the customer better.
Shailesh Mourya (00:23:54)
Yeah, I think things like these are helping very well, not just the e-commerce industry, but even for BFSI brands where they are leveraging the power of AI/ML to reduce frauds or do better product offering.
Vrinda Aggarwal (00:24:42)
In fact, BFSI is always the first mover with the high ticket value items and more of the liabilities for frauds and insurance burdens and all. Yeah. They are the first ones to adopt any kind of technology like this.
Shailesh Mourya (00:25:04)
Absolutely. So, Vrinda, to conclude this discussion, what are the top three things marketeers should keep in mind when they’re trying to utilize the power of AI/ML to boost their customer engagement rates and ultimately the conversion rate? Because that’s what the ultimate objective is.
Vrinda Aggarwal (00:25:15)
So, should — So, Shailesh, the basics for this is also the same as for getting a data-driven mindset for you as well. Start small, but consistent. So, don’t get bogged down by the fancy use cases, which is now available in — in most of the blog sites — websites, or, you know, just at the tip of your Google searches. But start with a known data set where you can make out inconsistencies or inaccurate results very fast, rather than something which is unknown for you. Design a model for a known problem or even an extension of the known problem. Plug back the results in the real world. And then with the results and the outcomes matching or not matching, the basis of prediction to the real world, plug back the real — real-world outcomes back into the model to improve upon them.
Shailesh Mourya (00:25:24)
OK.
Vrinda Aggarwal (00:25:29)
Efficiency to improve on the accuracy of the model
Shailesh Mourya (00:25:34)
So we’ll keep feeding data so that the system improves.
Vrinda Aggarwal (00:26:15)
You keep feeding — Exactly. Now the problem is if you start with the unknown data set and then you are trying to tackle a problem which is new. You will not even know whether the biases or the inconsistencies are coming in your data set or in the outcomes, and how to fine-tune that model. So, at least that is the approach that I have adopted while taking my learning back from the classroom to marketing.
Shailesh Mourya (00:26:32)
So first, one, you’re saying is start small, but be consistent. What are other two according to you?
Vrinda Aggarwal (00:26:46)
So, second is, cleanse and standardize the data which you’re using on a day-to-day basis. Because most of the companies, what they do, they start — for example, they start collecting the data in a particular set: with the change of the person in charge, that data model will change. Then another person will introduce their own formats. So, what happens at the end of the five years while the data is getting collected? From one to five years, the formats are not same, it’s not standardized. So, no model can run then on those five years of legacy data to give you a better outcome, it will always be in piecemeal, and if you try to go back and standardize it, it’s humanly not possible to do. So, pick up a standard — standardized format, a clean data set format, and follow it consistently. Minor modifications/additions are always welcome, but don’t change the entire KPI definition until there is — it’s a business limitation that you’re doing.
Shailesh Mourya (00:27:16)
Yeah. So, it is like using a single customer engagement platform, like Netcore, so that — I’m taking the liberty to talk about my company here — so — so that your data is not kept in silos and you’re getting a single view of the customer — of the data, which is stored in Netcore itself, and then you are able to run different campaigns as per customer need.
Vrinda Aggarwal (00:27:26)
Definitely. Or having very, very rigorous backup systems.
Shailesh Mourya (00:27:36)
Absolutely. So, what, what is the third one then?
Vrinda Aggarwal (00:28:07)
Third is to be patient. AI/ML is also not a magic wand that you plug in the data, you run the model and you’ll get amazing outcomes. It’s a slow and steady process where the model itself requires a lot of iterations and reiterations, and business sense on the top of it, is still required, for you to know how to leverage the outcomes into executable complaints or strategies, or on ground activity.
Shailesh Mourya (00:28:35)
Yeah, that’s, that’s great, Vrinda, awesome insights. It was great having you on the show, Vrinda. Thank you so much.
Vrinda Aggarwal (00:28:40)
Thanks, Shailesh. It was my pleasure.
Shailesh Mourya (00:28:41)
I’m sure—Yeah. I’m sure the audience will benefit greatly from all that you have shared with us today. The conversation has been very insightful. Folks, do subscribe or follow the Martechno Beat podcast and watch this page for more. Until next time! Thank you very much.
Vrinda Aggarwal (00:29:01)